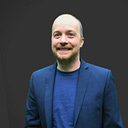
Discover more from Moving climate: a newsletter on migration and climate change
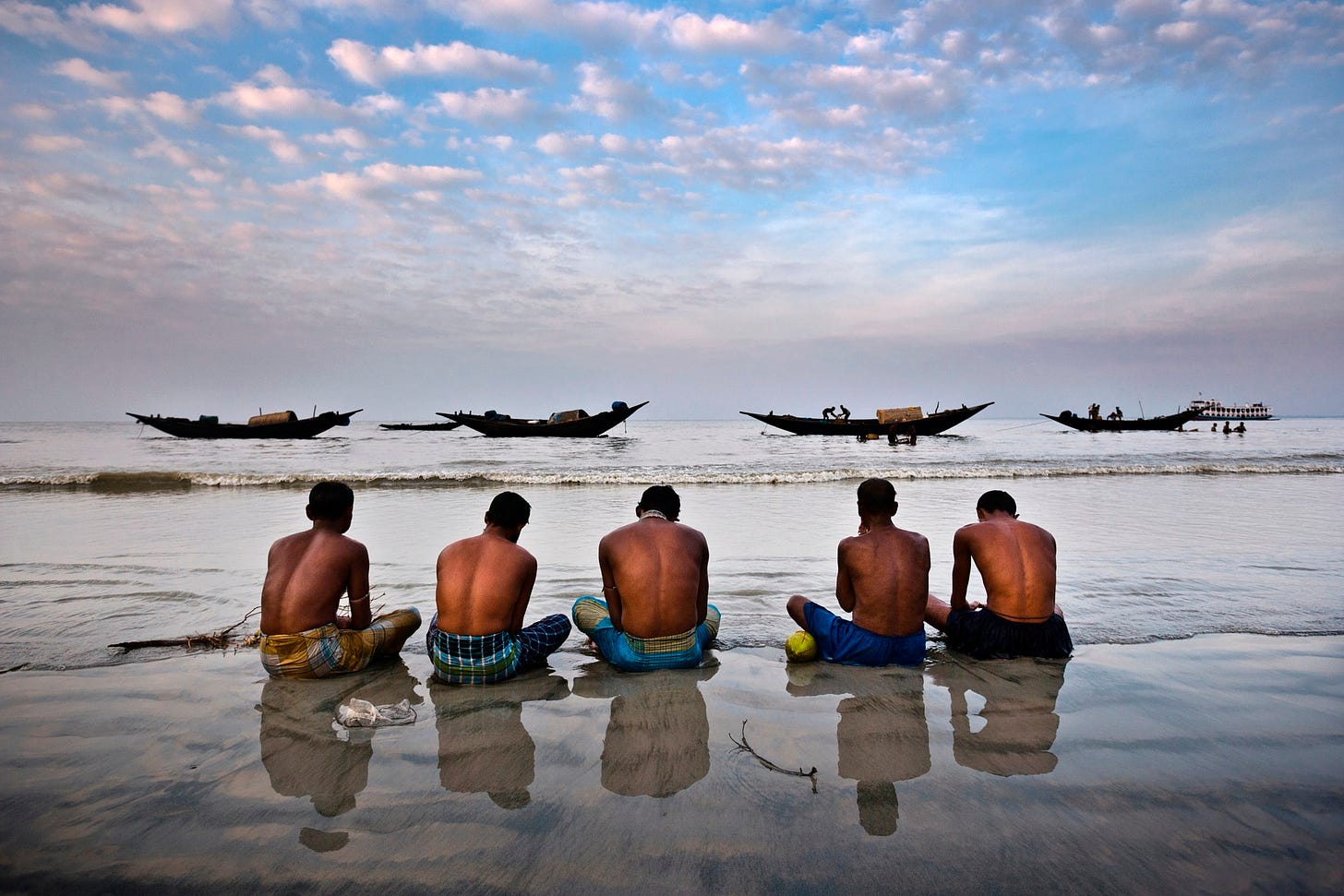
Last week, I dunked on a new report that repeated the notorious “1 billion climate refugees” figure. Some of you got in touch to ask why making accurate predictions about climate mobility is so difficult.
Luckily, there is a new(ish) paper that looks at how these predictions are made and why they’re not all they’re cracked up to be. The paper How well can we predict climate migration? A review of forecasting models, published in Frontiers in Climate, examines why it's so difficult. The authors review 30 different climate mobility forecasting models to understand what they can and can’t tell us about the future.
Tl;dr
The study concludes that predictive models are better than they used to be. But they are not a crystal ball. The predictive models we have are best viewed as tools for thinking about different possible futures rather than actually making reliable predictions.
A quick tour
It’s worth doing a quick tour of how these predictive models work…
One common (but heavily criticised) method is exposure models, which map out climate hazards like rising sea levels or droughts alongside population data to identify who may be at risk. Imagine a coastal city that’s increasingly vulnerable to rising sea levels. An exposure model could overlay sea level rise projections onto the city’s population distribution to identify neighbourhoods most at risk of flooding. These models were popularised by Norman Myers. During the 90s he used these methods to create a range of global predictions. These are still widely quoted in the media, but mostly dismissed by the accademic community.
Agent-based models simulate how personal factors like social networks and job opportunities influence migration decisions. In a rural farming area experiencing unpredicatable rainfall, you could use an agent-based model to simulate individual farmers’ decisions to migrate. It would consider factors like rainfall, livelihood options and social dynamics, giving insights into how rainfall might impact individual decisions to move or stay. Those individual decisions can then be aggregated to make broader predictions. This is exactly what these models attempt to do in rice growing areas of Thailand.
Gravity models use factors like population size and distance to project migration flows, adjusting for climate impacts. They assume that people are drawn towards desirable or survivable locations and flee from dangerous ones. For example, a gravity model could project how populations might redistribute across within a country based on population size, distances to safe locations, and climate impacts. The World Bank’s Groundswell model is probably the most sophisticated example of this kind.
Extrapolation models use historical data to find relationships between climate factors and migration patterns, then apply these insights to forecast future trends. By examining how specific climate events—such as flooding, soil degradation, or changes in rainfall—have influenced migration in the past, these models try to identify which areas and population groups are more likely to migrate under similar conditions in the future. This is exactly what these researchers did to make predictions about the impact of soil salinity on migration in Bangladesh.
Are they any good?
Non-climate factors are not in the mix
One major problem with many predictive models is that they don’t account for non-climate factors like family connections, economic factors, and conflict—all major forces in shaping current patterns of movement. For example, while social networks play a major role in influencing where and when people move, most models don’t incorporate them. Conflict, a critical driver of migration, is similarly underrepresented, with only one of the thirty models reviewed in the paper including it.
Imagine an area where both climate factors (e.g., drought) and other forces like poverty are prompting migration. Suppose you want to try to predict where people will move as drought intensifies and they seek alternative livelihoods. If your predictive model has no way of accounting for social connections in migration decisions, it will probably give you the wrong answer. A basic model would probably tell you that people will move to the nearest place they can find work. It will miss the fact that people are likely to follow friends or family members who have already moved, often to very specific neighbourhoods in large cities.
If conflict were to arise in the area, it might further accelerate migration or redirect it entirely. By leaving out these non-climate forces, most models risk oversimplifying migration patterns and underestimating the complexity of real-world migration responses to climate change.
Most models focus on slowly unfolding events, not rapid disasters
A major problem with climate mobility models is their tendency to focus on slow-onset changes, like sea level rise or gradual temperature increases. They overlook sudden-onset events like hurricanes, floods, or wildfires. Many models predict migration based on a single hazard or a small set of slow, predictable changes. But in the real, messy world, climate-linked mobility is driven by a mix of both slow and sudden changes.
Other “big stuff” will probably happen
A major problem for all models—no matter how sophisticated—is big future unknowns. Unforeseen events like pandemics, wars, or technological breakthroughs can drastically alter migration patterns.
Many models attempt to project as far into the future as 2100—76 years from now. Think back 76 years into the past to the year 1948. In 1948, could anyone have predicted the major drivers of migration and displacement over the next 76 years? Could anyone have foreseen the unravelling of European empires and the new patterns of migration it caused? Could anyone have predicted any of the major conflicts—Vietnam, the Second Congo War, the US and UK invasion of Iraq—that have collectively displaced millions of people? Could anyone have predicted extensive European integration and the new patterns of migration it created?
The point is that some of the biggest drivers of human movement are very unpredictable. The climate mobility models might be able to make predictions if all other factors—conflict, economy, technology—stayed the same. But things do happen. Things do change.
Where does this leave us?
The climate mobility models we currently have cannot produce accurate numerical predictions about the future. They cannot reliably tell us whether 50 million, 200 million, or 1 billion people will migrate because of climate change.
This doesn’t mean that the climate mobility models are useless. It means that we’re using them for the wrong things.
For example, they are useful for reducing uncertainty. They’re good at telling us a range of possible options when we’re looking at specific local situations. Suppose a city government needs to know how many people might leave the city due to heat stress over the next decade. Some of the models can provide a range of possible outcomes. That is a useful, usable insight.
They’re also good at helping policymakers think through different scenarios. How many people might move in a given area if rainfall decreases by 20% vs 50%? How might migration change in each of those cases if people are given various kinds of support? A model can’t give you an exact answer. But it can be a useful tool for thinking through complex future scenarios.
The problem is we try to use climate mobility models like a crystal ball to see into the future. But this isn’t what they’re for.
Subscribe to Moving climate: a newsletter on migration and climate change
Climate change holds the power to reshape patterns of migration and displacement. This is a newsletter about what that means.